About CHARMNET
Our Center is supported by the the Advanced Scientific Computing Research program of the U.S. Department of Energy.
Our Team
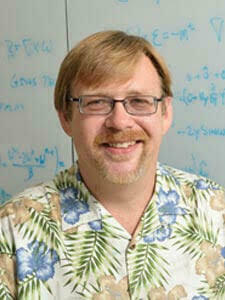
Andrew Christlieb currently serves as the Director of the Center for Hierarchical and Robust Modeling of Non-Equilibrium Transport (CHaRMNET), one of four Department of Energy Mathematical Multifaceted Integrated Capability Centers. In 2001, he received his PhD in Mathematics from the University of Wisconsin. After completing two post-docs at the University of Michigan (one in aerospace and one in mathematics), in 2006 he began his career at Michigan State University. In 2007, He was recognized by the Young Investigator Program (YIP) of the Air Force Office Of Scientific Research. From 2007-2011 he was an Intergovernmental Personnel Act (IPA) with Kirtland Air Force Base. His core research interests are scalable implicit algorithms, high-order structure/asymptotic preserving methods for both kinetic and fluid models, fast integral equations methods, particle methods, blended computing paradigms, and structure-preserving machine learning. His group has been recognized by the National Science Foundation, Air Force Office of Scientific Research, Office of Navel Research, and the Department of Energy for their work on high order methods and novel approaches to multi-scale problems in plasma and material science. Dr. Christlieb has graduated 22 PhD students and mentored 17 post-docs who have gone on to positions in industry, universities and the national labs. He is currently a University Foundation Professor of Mathematics at Michigan State University and from 2015-2021, Dr. Christlieb served as the founding chair of the Department of Computational Mathematics, Science and Engineering at Michigan State University.
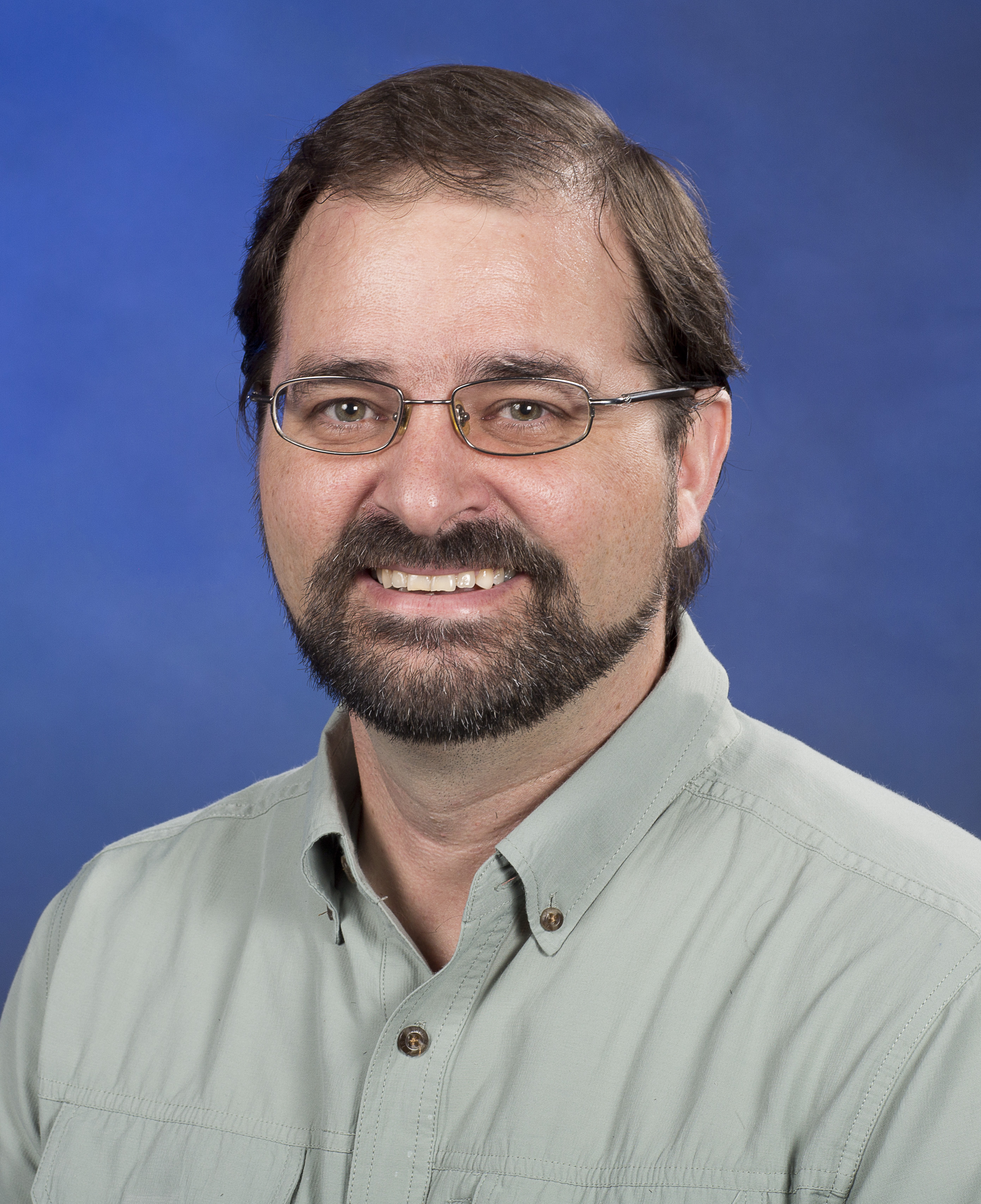
Luis Chacon is a Senior Scientist in the Applied Mathematics and Plasma Physics group in the Theoretical Division at Los Alamos National Laboratory and the co-Director of CHaRMNET. His research interests include the development of modern scalable multiscale algorithms for fluid and kinetic modeling of plasmas, with emphasis on implicit time integration, structure and asymptotic preservation, and spatial adaptivity. He is an E. O. Lawrence Award Laureate and a Fellow of the American Physical Society.
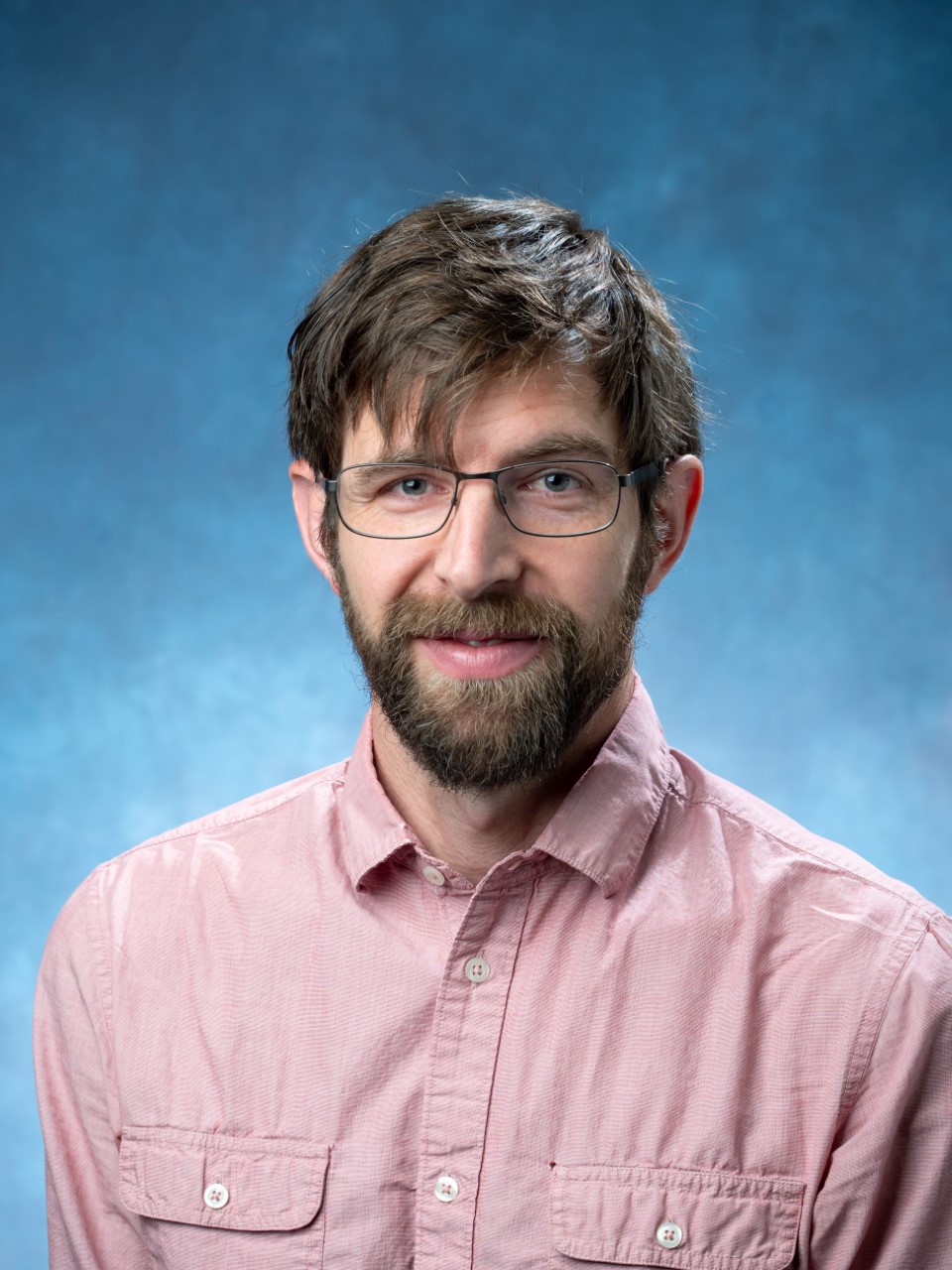
Stephen Becker is an associate professor in the Applied Mathematics department at the University of Colorado Boulder. Previously he was a Goldstine Postdoctoral fellow at IBM Research and a Fondation Scientifique et Mathematique de Paris postdoctoral fellow. He received his PhD in Applied Mathematics from Caltech in 2011, and bachelors in math and physics from Wesleyan University. His research interests are in optimization, machine learning, signal processing, imaging, inverse problems in quantum information, PDE-constrained optimization, and randomized numerical linear algebra.
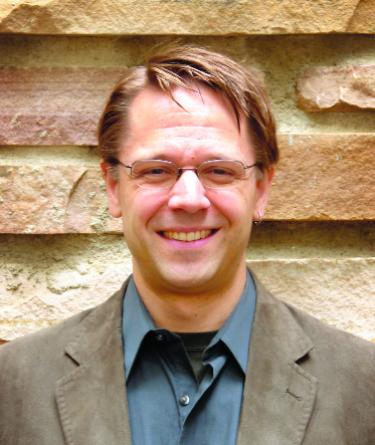
David Bortz is a Professor in the Department of Applied Math at the University of Colorado at Boulder. He earned his PhD in 2002 with H.T. Banks at North Carolina State University. He was then a postdoc at the University of Michigan in Mathematics and joined University of Colorado in 2006. The core of his research interests are in scientific computation methodologies for data-driven modeling and inverse problems at the intersection of applied math and statistics. His research has received support from NSF, NIH, DOE, DOD, and NVIDIA.
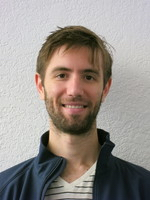
Joshua Burby is an assistant professor in the Department of Physics at University of Texas at Austin since January 2024. He was a Staff Scientist in the Applied Mathematics and Plasma Physics group at the Los Alamos National Laboratory. His research uses geometric methods to analyze the foundations of theoretical plasma dynamics. Josh earned his Ph.D. in plasma physics from Princeton University in 2015. He has been awarded distinguished postdoctoral fellowships by DOE FES (2015-2018), the Mathematical Sciences Research institute (2018), and the Los Alamos National Laboratory (2019-2022). He currently serves as Co-PI on a multi-institution ASCR project devoted to developing machine-learning based dynamical surrogates with stability guarantees.
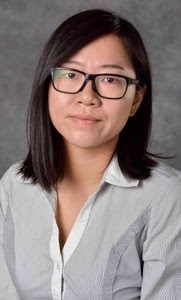
Yingda Cheng is a Professor at Department of Mathematics and an affliated faculty with CMDA (Computational Modeling and Data Analytics) program, Virginia Tech. Her specialty is in numerical methods of PDEs, in particular, discontinuous Galerkin methods and deterministic kinetic simulations. Recently, her group is devoted to developing reduced order models for complex kinetic transport using tools from machine learning, reduced order modeling and sparse grid techniques. Dr. Cheng received her B.S. degree from University of Science and Technology of China in 2003, and Ph.D. degree in Applied Mathematics from Brown University in 2007. She was a postdoc at the University of Texas at Austin from 2007 to 2011. She joined MSU in 2011, and has been assistant (2011-2016), associate (2016-2021) and full (2021-2023) professor in mathematics and Computational Mathematics, Science, and Engineering (CMSE). She moves to Virginia Tech as professor at Department of Mathematics since August 2023. She is a NSF CAREER award receipt (2015) and Simons Fellow in Mathematics (2018).
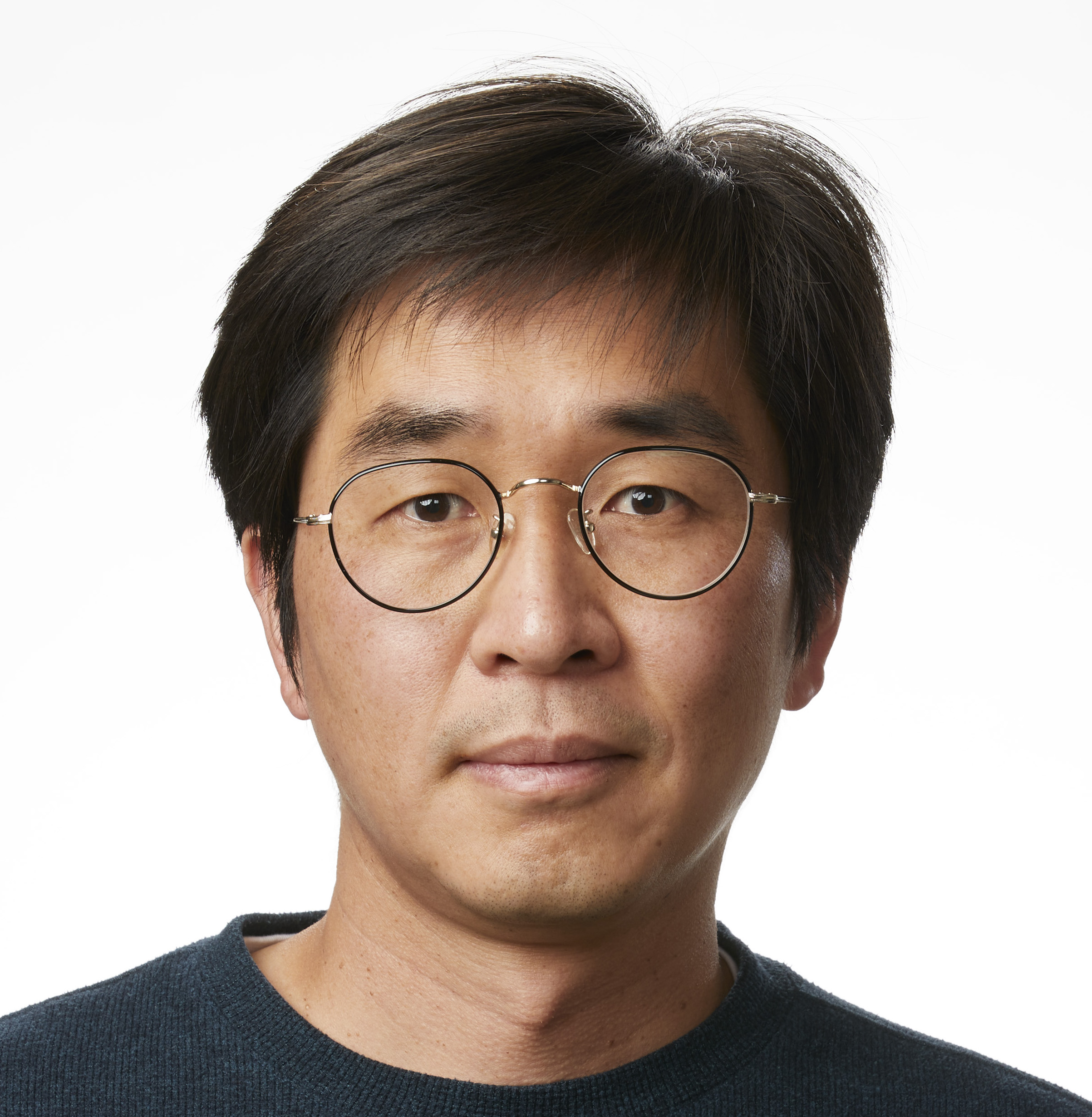
Youngsoo Choi is a research scientist in Center for Applied Scientific Computing at Lawrence Livermore National Laboratory. His research focuses on developing efficient data-driven reduced order models for physical simulations for time-sensitive decision-making multi-query problems, such as inverse problems, design optimization, and uncertainty quantification. He is currently leading data-driven reduced order model development team, with whom he developed the open-source code, libROM. libROM is a library for various reduced order modeling capabilities, e.g., physics-constrained projection-based reduced order models, dynamic mode decomposition, and physics-informed latent space dynamics discovery methods. He has earned his undergraduate degree for Civil and Environmental Engineering from Cornell University with applied mathematics as minor and his PhD degree for Computational and Mathematical Engineering from Stanford University. He was a postdoc in Sandia National Laboratory and Stanford University prior to joining LLNL in 2017.
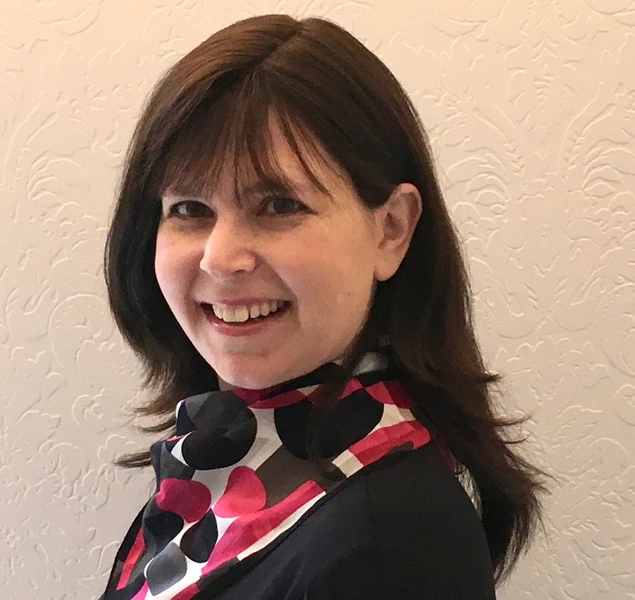
Sigal Gottlieb received her Ph.D. from Brown University in 1998 and in 1999 joined the Mathematics Department at UMass Dartmouth, where she us currently Chancellor Professor in the mathematics department. Professor Gottlieb is known internationally as an expert in strong-stability-preserving time discretizations and other schemes for hyperbolic equations. She has published two books and over 40 research papers in peer-reviewed journals of high caliber. Her papers have over 8000 citations, with her top two papers having over 2300 citations each. Her area of research is in computational and applied mathematics, and her work has been continually funded by the Air Force Office of Scientific Research (AFOSR) and the National Science Foundation (NSF). She is a Fellow of the Society of Industrial and Applied Mathematics and of the Association for Women in Mathematics. For four years (2017-2021) Professor Gottlieb was a Deputy Director at the NSF funded Institute for Computational and Experimental Research in Mathematics (ICERM). Dr. Gottlieb is founding director of the Center for Scientific Computing and Data Science Research, the hub for computational science research at UMassD and aims to support faculty doing computational research at UMassD, and promote internationally-recognized computational research that advances the fields of modern applied science, data-driven and data science algorithms. She has led several successful equipment proposals for large-scale computing clusters that support the research of CSCDR affiliates. In related activities, she was instrumental in the development of new academic programs, including the EAS doctoral program and the Data Science BS and MS programs.
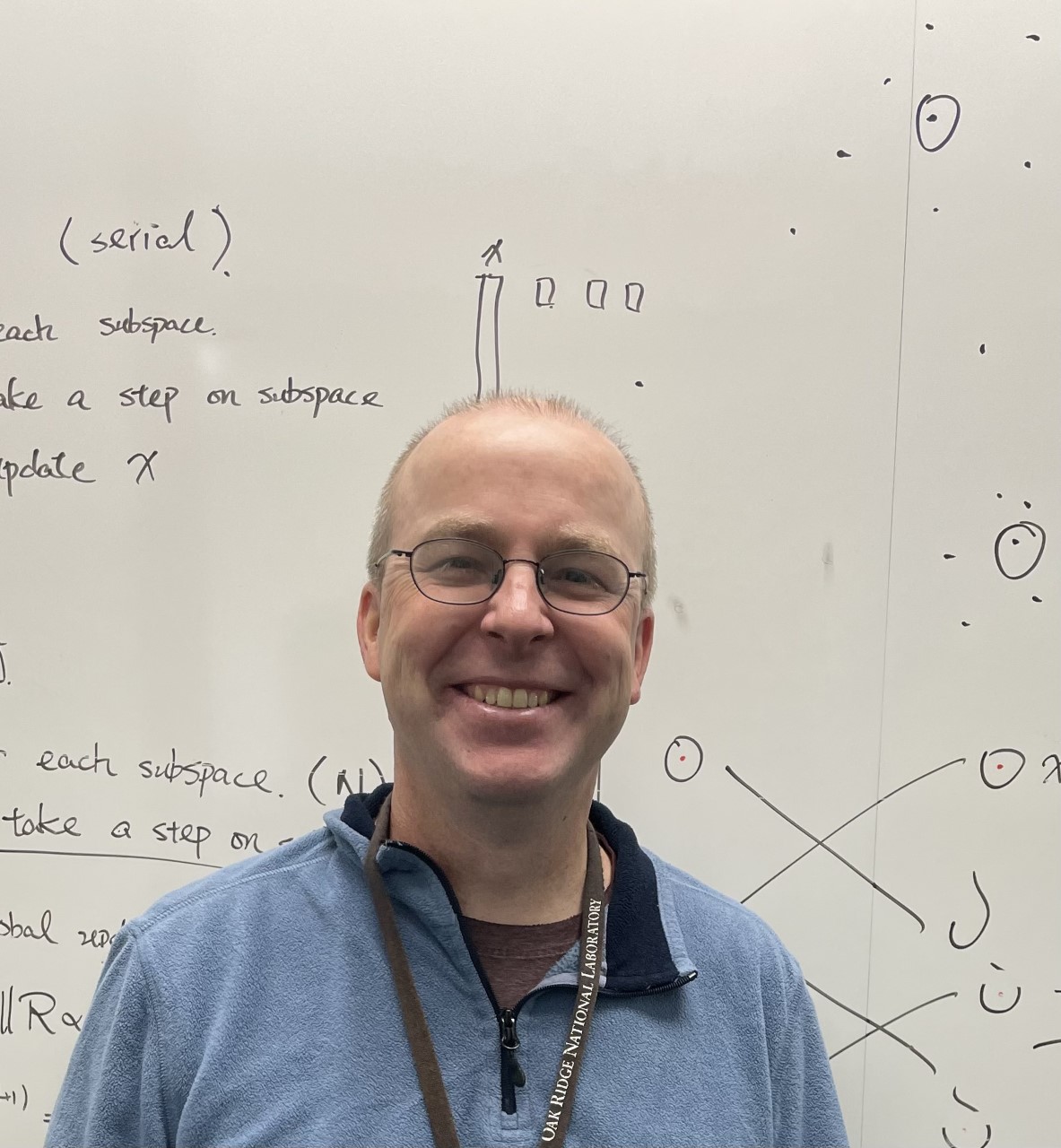
Cory Hauck is an applied mathematician and group leader of the Multiscale Methods and Dynamics Group at Oak Ridge National Laboratory. He received his Ph.D in Applied Mathematics from the University of Maryland in 2006, and before coming to Oak Ridge, sat in the Center for Nonlinear Studies at Los Alamos National Laboratory as a postdoc in the Computational Physics and Methods Group . Dr. Hauck is interested in various aspects of numerical analysis, computational physics, scientific computing, and mathematical tools for scientific data. His primary research area is the development of numerical methods for kinetic equations and related models. These models are characteristic by multiscale phenomena, and their simulation requires advanced mathematical tools in modeling and computation.
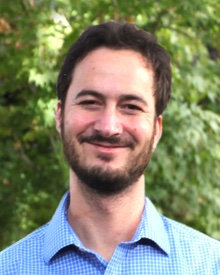
Terry Haut is a Staff Scientist in Center for Applied Scientific Computing at Lawrence Livermore National Laboratory (LLNL), and is a member of LLNL’s Deterministic Transport Team. His research focuses on developing multiscale methods and high-order finite element methods for kinetic equations, as well multiscale parallel-in-time methods for geophysical fluid applications.
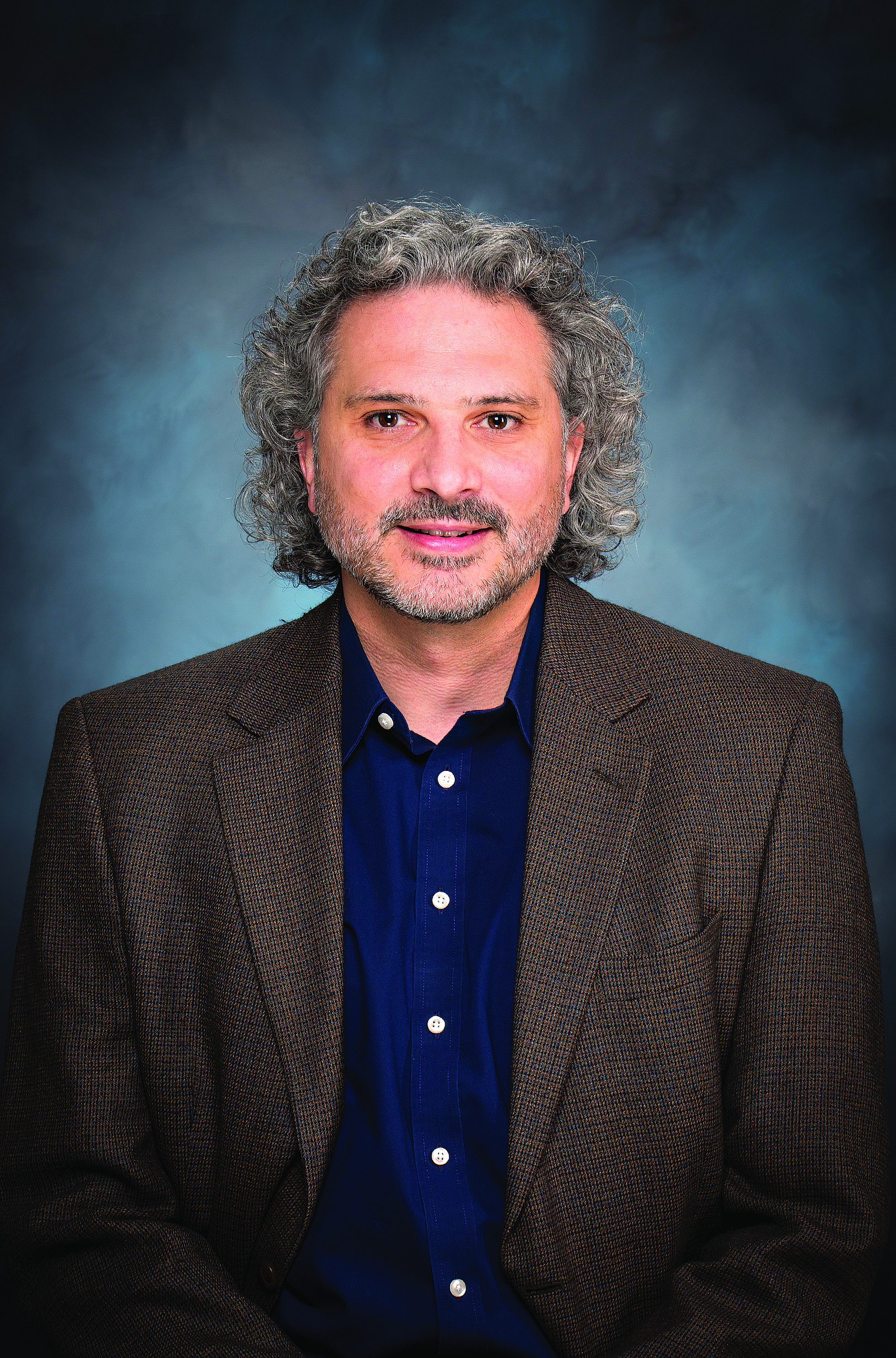
Jeff Hittinger is the Director of the Center for Applied Scientific Computing (CASC) at Lawrence Livermore National Laboratory (LLNL), the nexus of research in computational mathematics, computer science, and data science in the Computation Directorate. Prior to this, he was the Group Leader for and a Computational Scientist in the Scientific Computing Group in CASC. Jeff works primarily on the application of advanced discretization methods and techniques to plasma physics simulation, and he led LLNL’s Variable Precision Computing Strategic Initiative project. His research interests include computational plasma physics, computational fluid dynamics, higher-order finite-difference and finite-volume methods, parallel computing, adaptive mesh refinement, error estimation, and variable precision computing. Jeff joined CASC in October 2000 as a postdoctoral researcher. Prior to this, he attended the University of Michigan where he received his Ph.D. in Aerospace Engineering and Scientific Computing (2000), his M.S. in Mathematics (1997), and his M.S.E. in Aerospace Engineering (1994). Jeff received his B.S. in Mechanical Engineering from Lehigh University (1993).
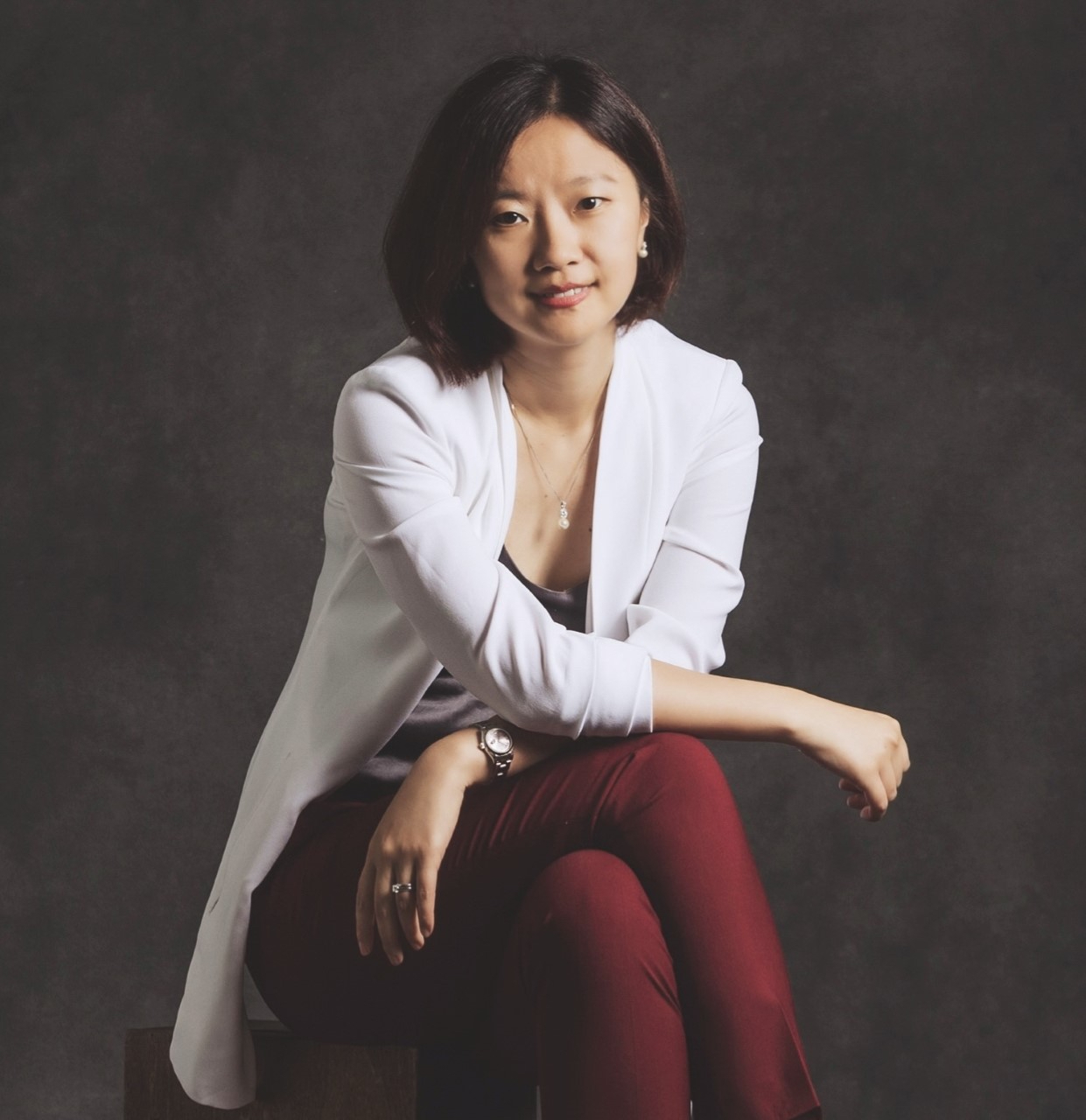
Jingwei Hu is an associate professor in the Department of Applied Mathematics at the University of Washington. She also holds an adjunct professorship in the William E. Boeing Department of Aeronautics & Astronautics. Her general research interests lie in numerical analysis and scientific computing, in particular, development of numerical methods for multiscale kinetic equations arising in various science and engineering applications. She serves on the editorial boards of a few mathematical journals including La Matematica and Kinetic & Related Models..
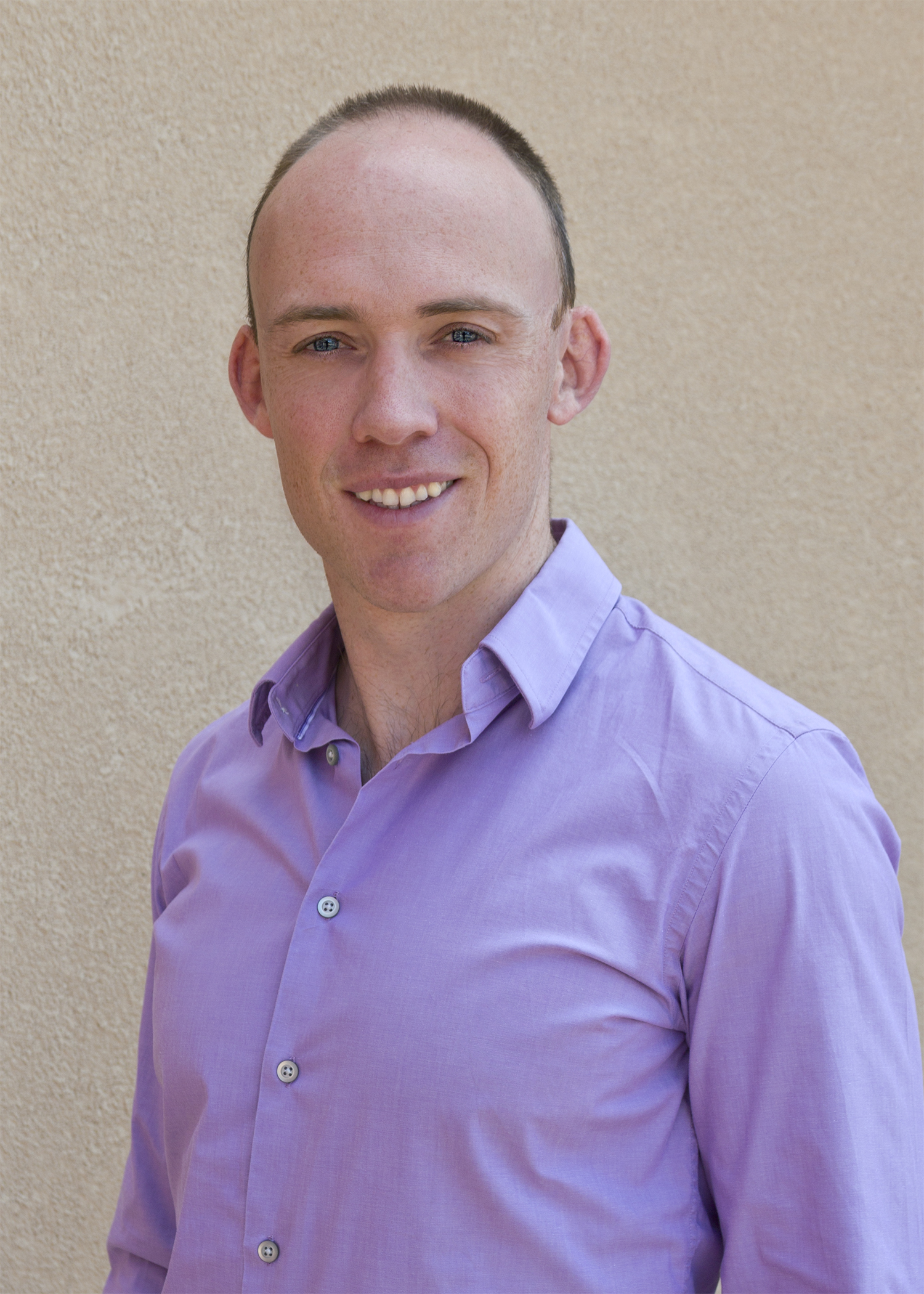
John Jakeman is a Principal Member of Technical Staff at Sandia National Laboratories (SNL) and the co-institutional PI of CHaRMNET at SNL. He received his Ph.D. in mathematics from the Australian National University, Canberra, Australia in 2011. John is the author of over 50 articles that have covered topics including surrogate modeling, experimental design, uncertainty quantification and multi-fidelity modeling. He is the lead developer and founder of the Python software Package PyApprox for uncertainty quantification, experimental design and scientific machine learning.
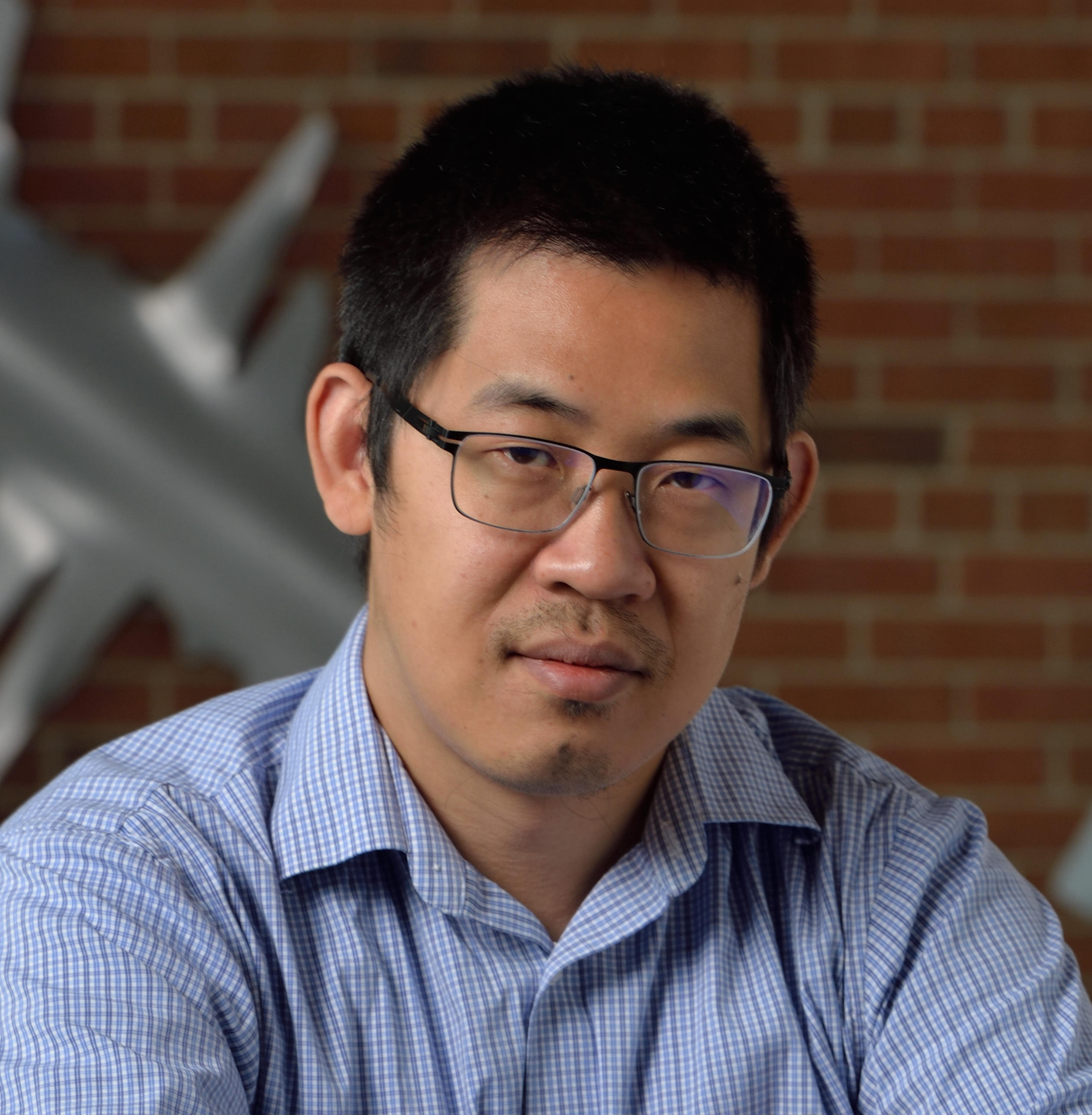
Huan Lei is an Assistant Professor in the Department of Computational Mathematics, Science, and Engineering with a joint position in the Department of Statistics and Probability at Michigan State University. He received his Ph.D. degree in Applied Mathematics from Brown University in 2012. He was a post-doc (2013-2015) and scientist (2015-2019) at Pacific Northwest National Laboratory, and joined Michigan State University in 2019. His research interest is in applied mathematics and scientific computing, in particular, multi-scale modeling and model reduction with applications to fluid physics, materials science, and biophysics. Currently, his group is devoted to developing data-driven algorithms to learn reliable and physics-interpretable models of meso-scale transport, assembly, and transition processes directly from the micro-scale first-principle-based descriptions.
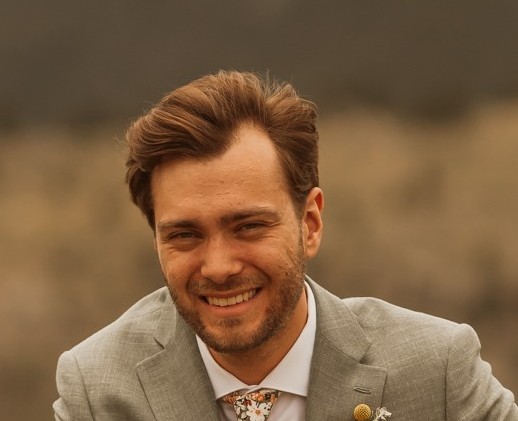
Daniel Messenger is a Research Associate in the Applied Mathematics Department at the University of Colorado Boulder. He will begin a Director's Postdoctoral Fellowship in the T-5 Group in the Theoretical Division at Los Alamos National Laboratory in Fall 2024. Daniel received his MSc in Applied Mathematics from Simon Fraser University in 2019, and his PhD in Applied Mathematics from University of Colorado Boulder in 2022. His research concerns data-driven modeling with an emphasis on weak-form methods, and interacting particle systems, with applications including plasma physics, cell migration, and ecology.
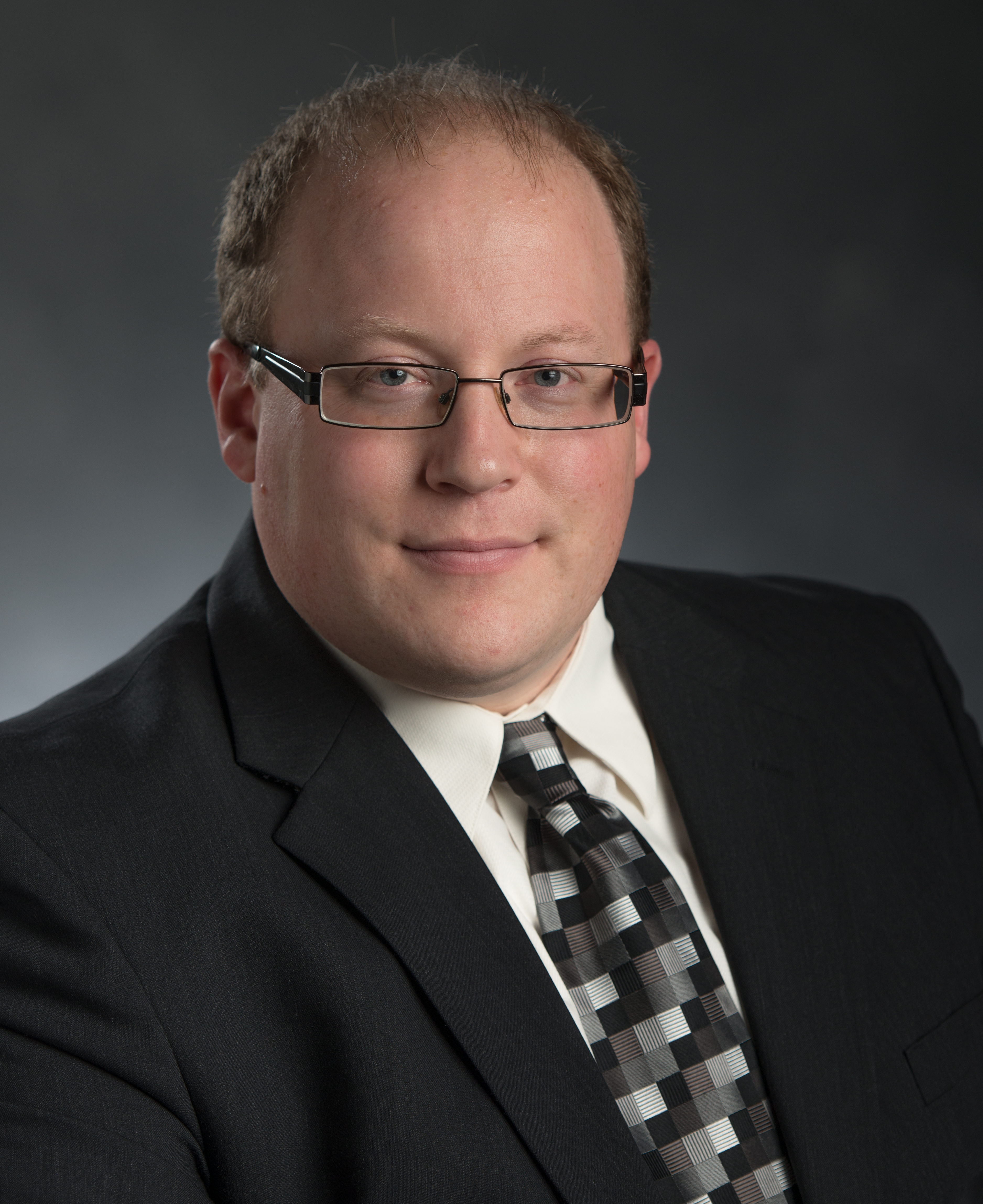
Brian O'Shea is a computational and theoretical astrophysicist studying cosmological structure formation, including galaxy formation, the behavior of the hot, diffuse plasma in the intergalactic medium and within galaxy clusters, and (more recently) terrestrial high energy density plasma physics phenomena. He is also a co-author of several open source software tools including the Enzo, Enzo-E, and Athena-PK codes, an expert in high performance computing, and an advocate for open-source computing and open-source science. He received his B.S. in Engineering Physics at the University of Illinois in Urbana-Champaign (UIUC) in 2000, and his PhD in physics from UIUC in 2005. Following that, he was a Director's Postdoctoral Fellow at Los Alamos National Laboratory, with a joint appointment between the Theoretical Astrophysics Group and the Applied Physics Division. Since 2008, he has been a member of the faculty at Michigan State University, with a joint appointment between the Department of Computational Mathematics, Science, and Engineering (2015-present), the Department of Physics and Astronomy (2008-present), and the National Superconducting Cyclotron Laboratory (2014-present). From 2008-2015, Dr. O'Shea was a member of Lyman Briggs College. He has authored or co-authored over 100 peer-reviewed journal articles in astrophysics, computer science, and education research journals, and has received a variety of awards for his teaching and public outreach efforts. In 2016, he became a Fellow of the American Physical Society, and in 2019 he became the Director of MSU's Institute for Cyber-Enabled Research.
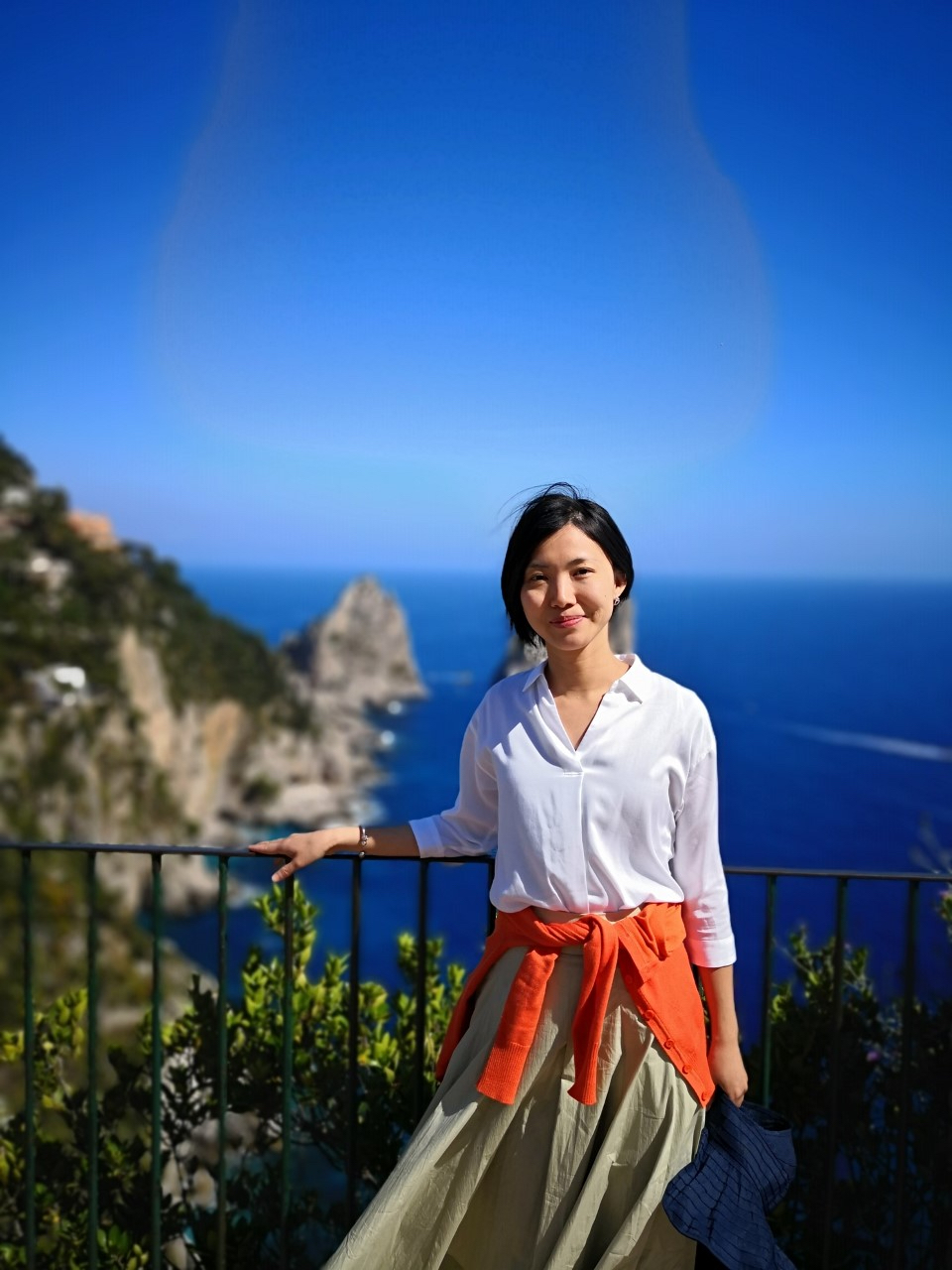
Jingmei Qiu is a Professor of Mathematics at the University of Delaware. Her general area of research is numerical analysis and scientific computing. Her specialty area is in the design, analysis and application of high order structure preserving computational algorithms, for complex systems that involve multi-scale and multi-physics features and are of high dimensional nature. She has been working on high order numerical methods for fluid, kinetic and multi-scale models. Recently she is interested in Eulerian-Lagrangian high order approaches, and low rank approximations to high dimensional nonlinear dynamics. Her methodology has been applied to astronomy, physics, and engineering problems.
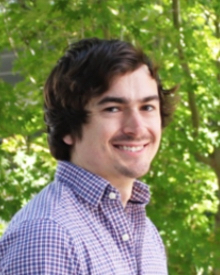
Lee Ricketson is a Staff Scientist in the Center for Applied Scientific Computing at Lawrence Livermore National Laboratory. His research develops novel algorithms for simulating kinetic plasma behavior, focusing in particular on overcoming the curse of dimensionality and multiple time-scales present in such systems. He earned his Ph.D. in mathematics at UCLA in 2014, where he was awarded the outstanding dissertation prize from the Pacific Journal of Mathematics. Before joining Livermore, he earned the Cathleen Morawetz Fellowship Award for outstanding technical postdoctoral achievement at New York University’s Courant Institute of Mathematical Sciences.
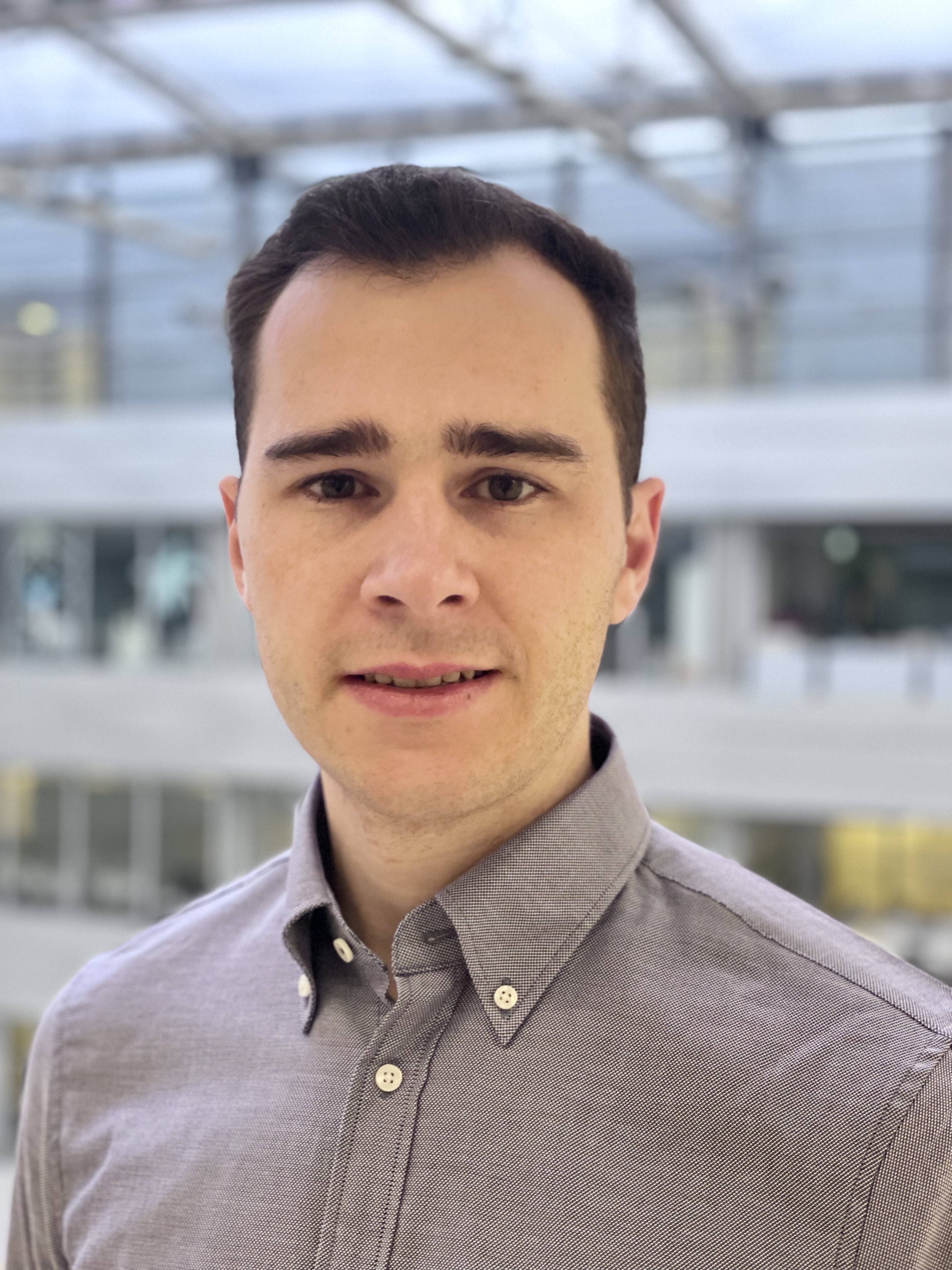
Steffen Schotthöfer is the current Householder Fellow at Oak Ridge National Laboratory. He received his Ph.D in Mathematics from Karlsruhe Institute of Technology, Germany, in May 2023. His dissertation considers synergies between numerical methods for kinetic equations and neural networks. In 2022 he was a visiting researcher at ORNL. Prior to his PhD, Steffen obtained his Master's Degree in Applied Mathematics and Computer Science from TU Kaiserslautern, Germany, and NTNU Trondheim, Norway in 2020, and his Bachelor's Degree in Mathematics and Electrical Engineering from TU Kaiserslautern in 2017. Steffen's research interests lie in the development of Dynamical Low-Rank Approximation methods for modern, large-scale machine learning and their application as well as data-driven surrogate methods for kinetic equations.
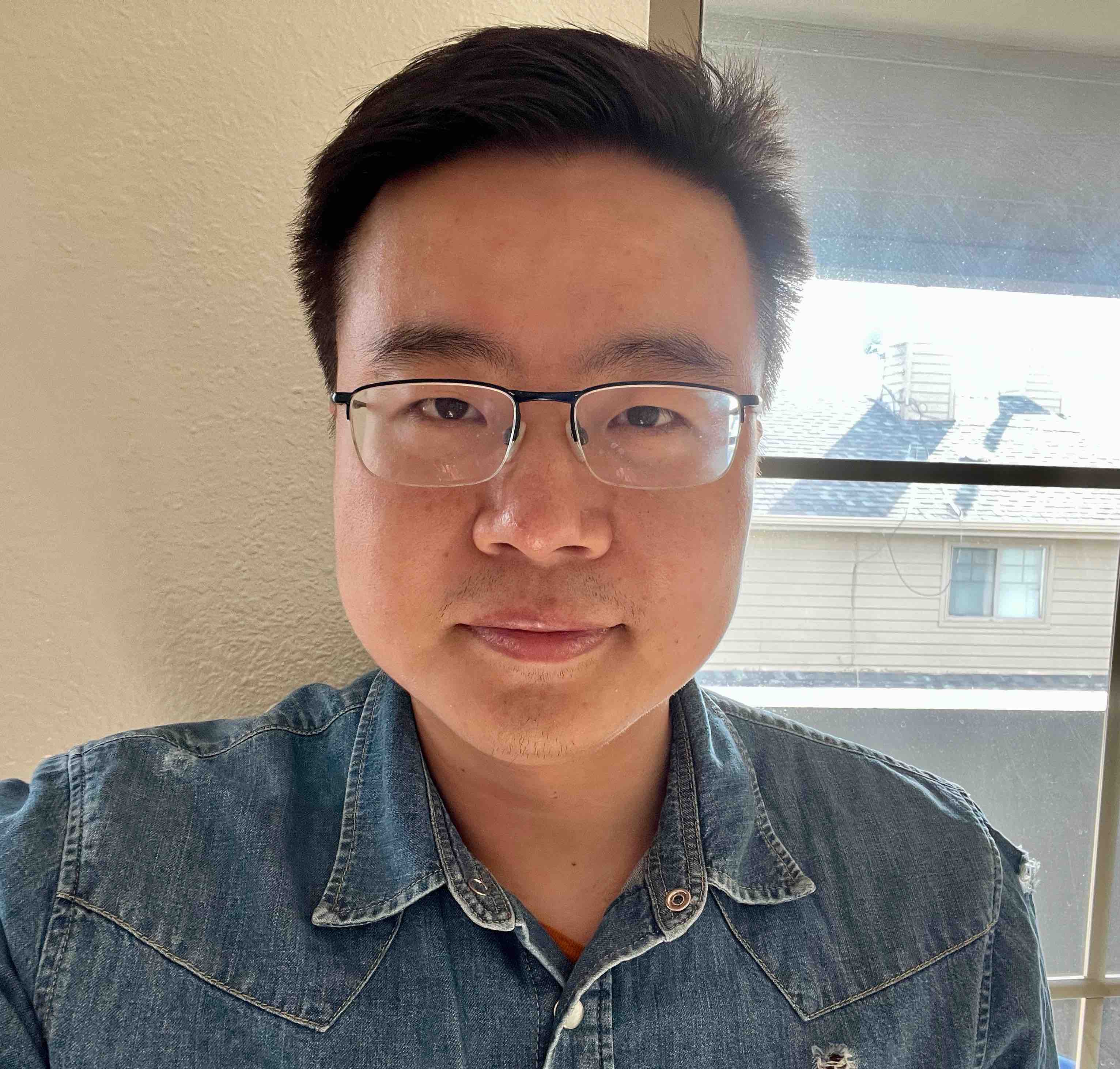
Qi Tang has been an assistant professor in School of Computational Science and Engineering at Georgia Institute of Technology since August 2024. He was a Staff Scientist in the Applied Mathematics and Plasma Physics Group in the Theoretical Division at Los Alamos National Laboratory. He received his PhD in applied math from Michigan State University in 2015 and BS in math & applied math from Zhejiang University in 2010. Before joining LANL in 2018 he was the Eliza Ricketts Foundation Postdoctoral Fellow at Rensselaer Polytechnic Institute. His research interests include: MHD, kinetic modeling, scalable numerical algorithms, multi-physics and multi-scale problems, and scientific machine learning. He is also a member of the TDS SciDAC.
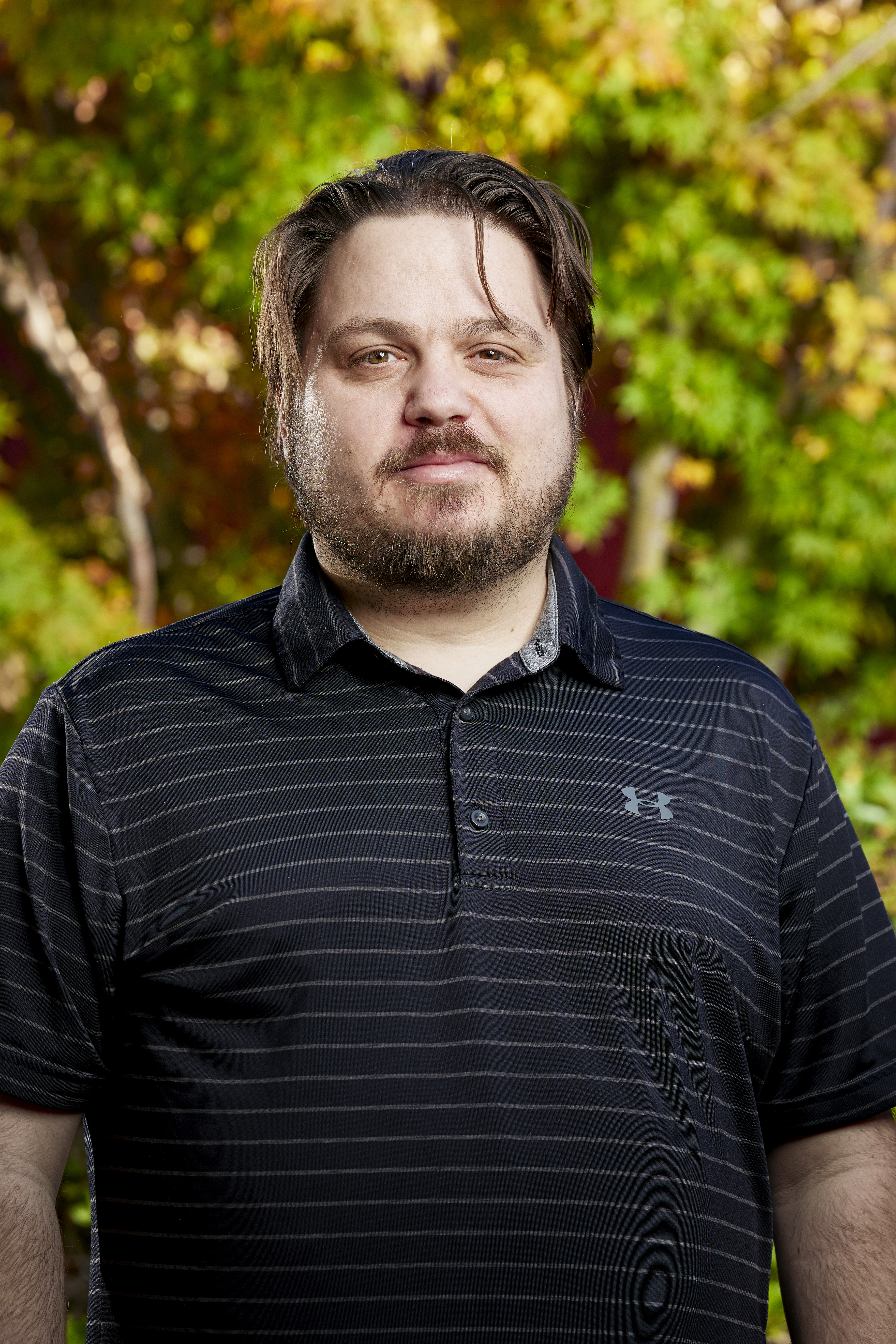
Paul Tranquilli is a Member of Technical Staff at the Center for Applied Scientific Computing at Lawrence Livermore National Laboratory. He received his PhD from Virginia Tech in 2016. His research interests include advanced time integration methods for large systems of differential equations, and the efficient simulation of multiscale kinetic systems.
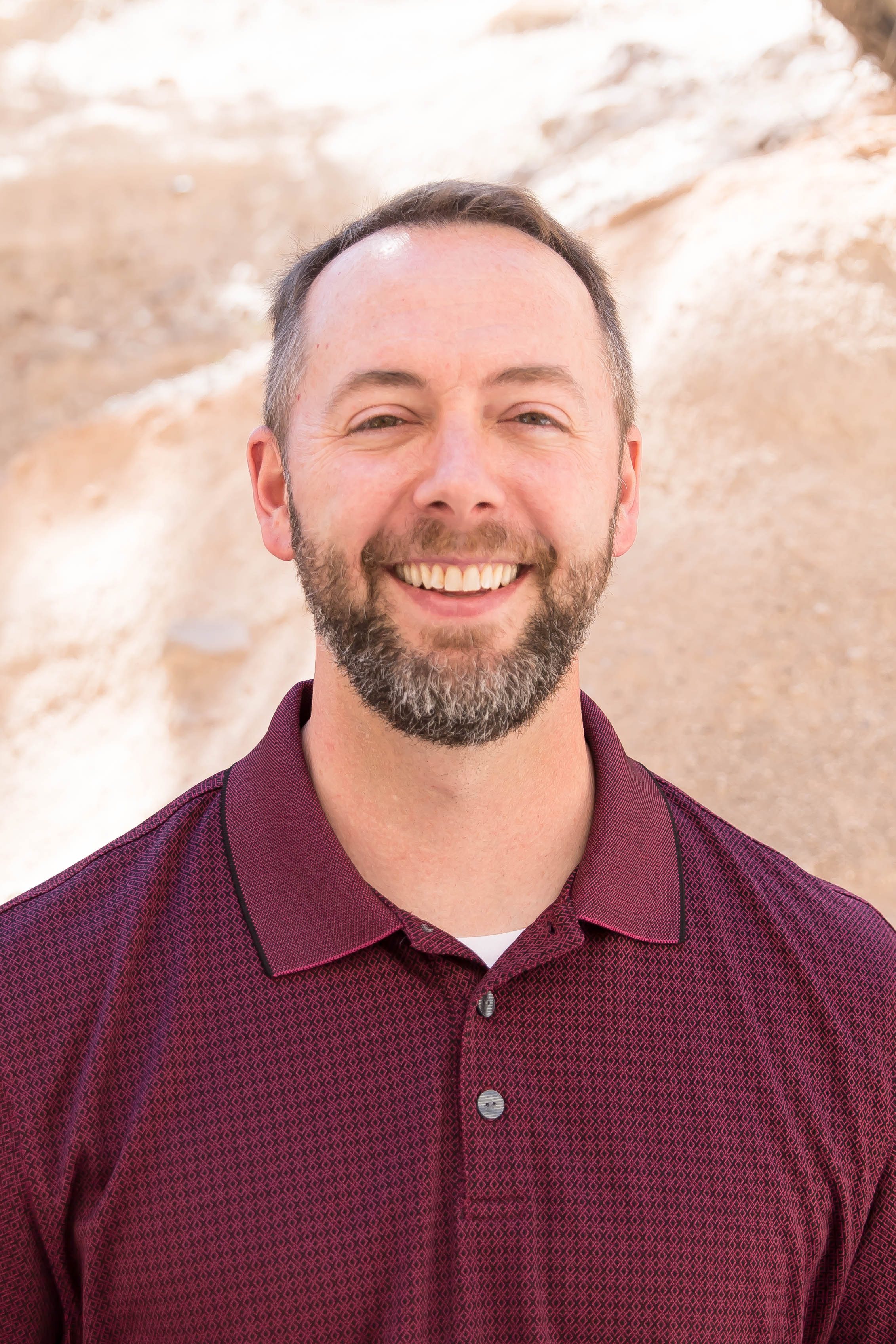
Tim Wildey is a Principal Member of Technical Staff in the Scientific Machine Learning Department at Sandia National Labs. He earned his PhD from Colorado State University in 2007 on adjoint-based a posteriori error estimation for coupled multi-physics applications and held the ICES postdoctoral fellowship at the University of Texas at Austin from 2007-2010 working in the Center for Subsurface Modeling on uncertainty quantification, multi-scale discretizations and multi-level preconditioners for coupled flow and mechanics in porous media. Since joining Sandia in 2011, he has worked on error estimation for surrogate models, physics-compatible and DG discretizations, multi-scale and multi-resolution hybridizable DG methods, Bayesian and data-consistent inversion methods, optimal experimental design, extreme-scale modeling and simulation for multi-scale formulations, developing algorithms for heterogeneous computational architectures, data-compression for memory-bound applications, and uncertainty quantification for scientific machine learning.